Under the strict prevention and control measures across the country, the new coronary pneumonia epidemic is under initial control. In order to prevent the outbreak of the epidemic again, people with asymptomatic infections and their risk of transmission are receiving more and more attention. From April 1, the National Health and Health Commission listed asymptomatic infected persons in the content of the epidemic situation notification, and adopted measures such as increased screening and expanded testing to strengthen management.
div> After expanding the detection range, the probability of false positives in the test results will increase accordingly and needs attention. This article introduces the phenomenon that the positive test results are distorted under a wide range of tests.
div> Suppose there are 10,000 people in society, and the probability of being infected is one in 10,000, that is, about one person is infected. The detection technology is very accurate but also has a small error, which is about 1 in 10,000, that is, one error may occur in 10,000 tests. Due to the existence of asymptomatic infections, this 10,000 people needs full coverage testing. Then, when the test is completed, how many positive samples are detected?
div> For the real infected person, the probability of being tested positive is very high, 99999/10000. For the other 9999 non-infected people, although the probability of each person being tested positive is very low, but because the detection range is too large, there will be about one “unlucky” non-infected person who is detected because of the 1 / 10,000th error Is positive (ie false positive). Therefore, there will be a total of about two people who test positive, including the one who is actually infected and the one who is mistested.
div> And we ca n’t tell which one is the actual infected person and which is the non-infected person who is mistested. So, if a person is tested positive, what is the probability that he will actually be infected? Since there were about two positive test results in total, he was either the actual infected person or the non-infected person who was mistested. Therefore, for a positive test result, we only have one-half sure that he is a real infected person, although the detection error rate is only one-ten-thousandth.
div> It can be seen that there are two reasons for the distortion of the positive test results: one is that the proportion of truly infected people in the population is low, they are usually tested as positive, but The number is small; the second is that the detection range is too large, although the detection error rate is very low, but there are too many people who are detected, and there are always a few people who are mistakenly tested as positive. Therefore, the proportion of samples that are falsely detected as positive to the total number of positive samples will be relatively high, resulting in distorted results.
div> The above analysis can provide the following enlightenment for the prevention and control of asymptomatic infected persons:
div> 1. The detection range for asymptomatic infected persons cannot be too wide, because the wider the detection range, the greater the degree of distortion of positive results. The guidance of the National Health and Health Commission is “expanding the detection scope to close contacts, key areas and key populations of found cases and asymptomatic infected persons” is very appropriate.
div> 2. After expanding the detection range, the objective existence of “false positives” should be fully paid attention to. It is recommended not to directly use a positive result as the basis for an asymptomatic infected person, but to confirm it as an asymptomatic infected person after repeated tests are positive.
div> 3. From the perspective of strict prevention and control, people who have a positive result can be isolated. However, such people must be quarantined as suspected cases, that is, single and single room isolation to avoid cross infection.
div> The method used in this article is called Bayesian analysis in probability theory. Interested readers may wish to refer to “Probability Theory and Mathematical Statistics (4th Edition)” by Sheng Su, Xie Shiqian, and Pan Chengyi (Higher Education Press, 2008) for more information. Of course, if you still think this “Probability Theory and Mathematical Statistics” is too professional, it is recommended to read the “What Statistics Are About Me-Minimalism in Life” by Japanese author Dr. Hiroshima Kojima Statistics strong> (Beijing Times Chinese Document Bureau, 2018).
div>
div> Epidemic prevention and control is a systematic project involving many disciplines, and various factors need to be considered comprehensively. This article only reminds of the phenomenon that positive results will be distorted after expanded range detection from the perspective of probability analysis.
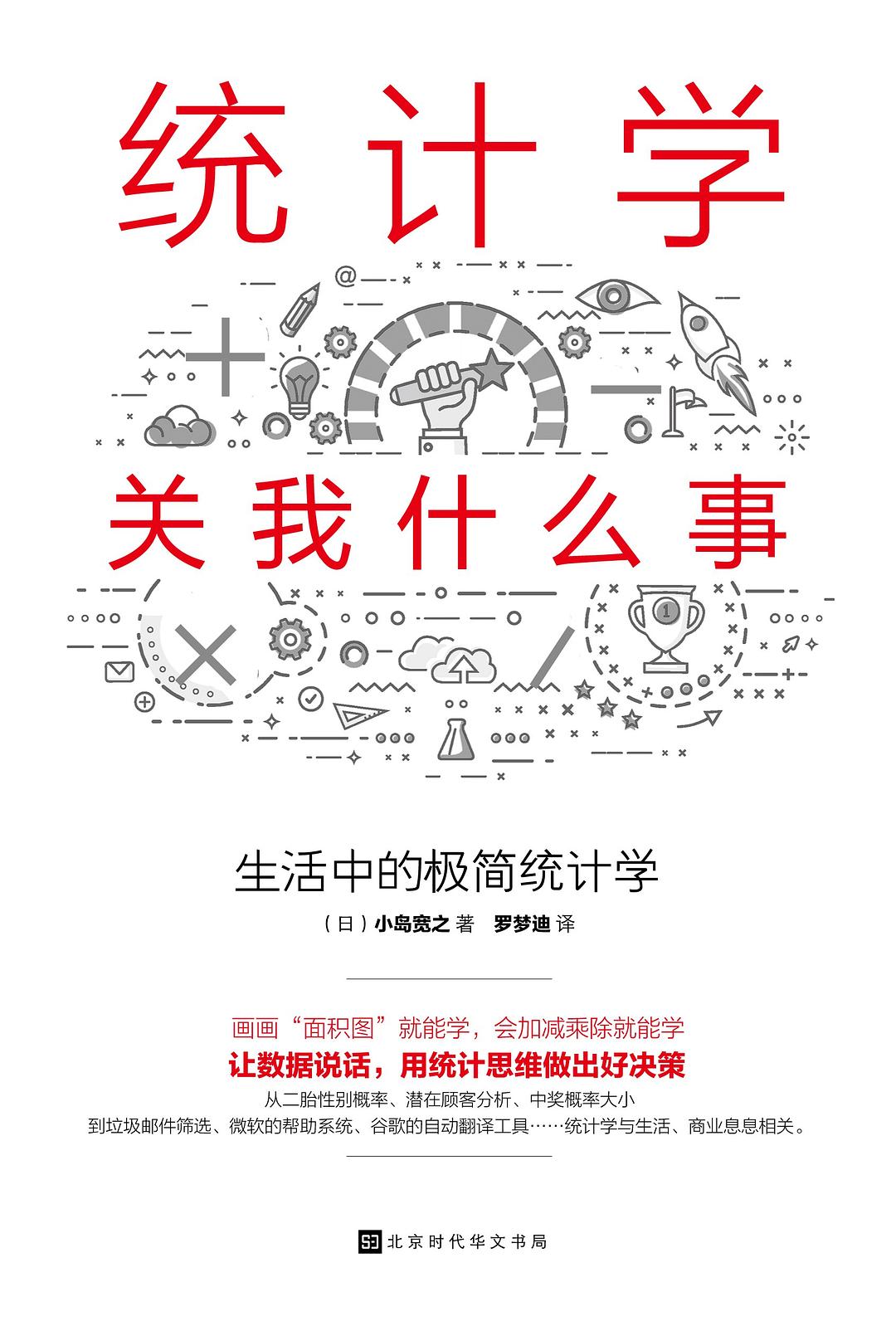
div> (Author Yu Tongkui is an associate professor at the School of Computer and Information Science, Southwest University) div>